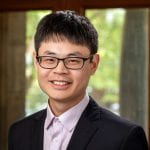
Speaker: Dr. Lu Lu – Yale University
Date: Sep 20, 2024; Time: 2:30 PM Location: PWEB 175
Abstract: As an emerging paradigm in scientific machine learning, deep neural operators pioneered by us can learn nonlinear operators of complex dynamic systems via neural networks. In this talk, I will present the deep operator network (DeepONet) to learn various operators that represent deterministic and stochastic differential equations. I will also present several extensions of DeepONet, such as DeepM&Mnet for multiphysics problems, DeepONet with proper orthogonal decomposition or Fourier decoder layers, MIONet for multiple-input operators, and multifidelity DeepONet. I will demonstrate the effectiveness of DeepONet and its extensions to diverse multiphysics and multiscale problems, such as bubble growth dynamics, high-speed boundary layers, electroconvection, hypersonics, geological carbon sequestration, full waveform inversion, and astrophysics. Deep learning models are usually limited to interpolation scenarios, and I will quantify the extrapolation complexity and develop a complete workflow to address the challenge of extrapolation for deep neural operators. Moreover, I will present the first operator learning method that only requires one PDE solution, i.e., one-shot learning, by introducing a new concept of local solution operator based on the principle of locality of PDEs.
Biographical Sketch: Dr. Lu Lu is an Assistant Professor in the Department of Statistics and Data Science at Yale University. Prior to joining Yale, he was an Assistant Professor in the Department of Chemical and Biomolecular Engineering at University of Pennsylvania from 2021 to 2023, and an Applied Mathematics Instructor in the Department of Mathematics at Massachusetts Institute of Technology from 2020 to 2021. He obtained his Ph.D. degree in Applied Mathematics at Brown University in 2020, master’s degrees in Engineering, Applied Mathematics, and Computer Science at Brown University, and bachelor’s degrees in Mechanical Engineering, Economics, and Computer Science at Tsinghua University in 2013. His current research interest lies in scientific machine learning, including theory, algorithms, software, and its applications to engineering, physical, and biological problems. His broad research interests focus on multiscale modeling and high performance computing for physical and biological systems. He has received the 2022 U.S. Department of Energy Early Career Award, and 2020 Joukowsky Family Foundation Outstanding Dissertation Award of Brown University. He is also an action editor of Journal of Machine Learning.